Surfacing the Unexpected: Improvements in Meteorological Modelling
Jessica Rumbelow
Co-founder & CEO
In a recent collaboration with the National Center for Atmospheric Research (NCAR)—a leading institution sponsored by the U.S. National Science Foundation—we applied the Discovery Engine to one of the most complex and dynamic systems on Earth: the coupled ocean-atmosphere boundary layer.
Using data collected from high-resolution floating instrument platforms, the study explored vertical profiles of ocean-atmosphere interactions. The dataset captured how atmospheric and oceanic variables—such as temperature, wind, and humidity—change with height above the sea surface, offering insights into the layered structure and dynamics of the boundary layer. The data contained key meteorological and oceanographic variables, including wind speed, temperature, pressure, humidity, wave characteristics, and crucial surface fluxes like sensible heat flux and friction velocity. (A 'flux' refers to the rate at which a physical quantity—such as heat or momentum—is transferred through a surface. Surface fluxes are essential for understanding how energy and motion move between the ocean and atmosphere.)
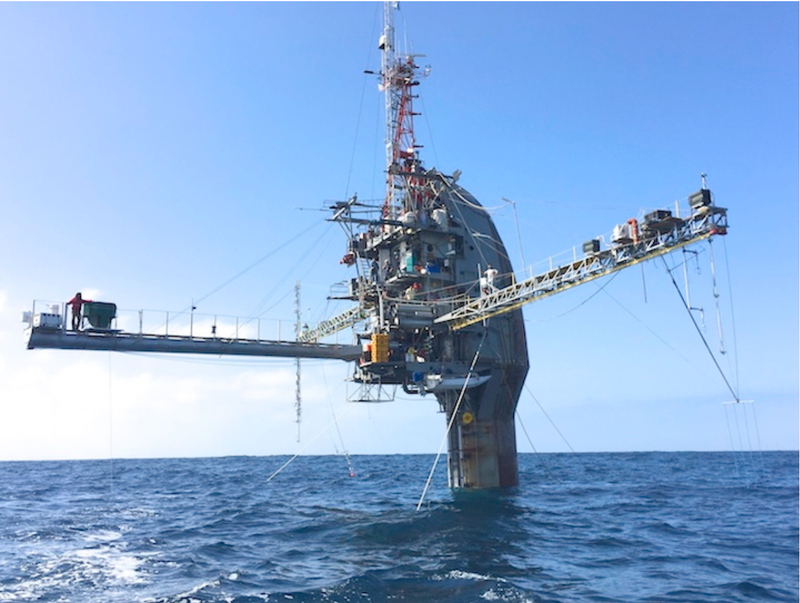
Challenging the Surface Layer Hypothesis
Heat flux refers to the rate at which heat energy is transferred vertically through the atmosphere. This transfer occurs primarily via turbulence—swirling motions that carry heat from warmer to cooler regions. Momentum flux, often represented by a quantity called friction velocity, describes the vertical transfer of wind momentum. In simpler terms, it's a measure of how surface friction affects the flow of air, which has critical implications for wind patterns and weather system development.
One foundational assumption in weather and climate models is the Surface Layer Hypothesis. Under unstable conditions (when greater mixing of air is assumed to occur), this hypothesis predicts that both heat flux and momentum flux remain nearly constant (within 10%) with height—implying that their gradients (how their values change as height increases) should be close to zero.
The Discovery Engine autonomously found many cases in the data where this does not hold true – an extremely important finding, as the Surface Layer Hypothesis underpins much of atmospheric modelling. When these assumptions break down, it compromises the accuracy of forecasts and simulations.
When forecasts are inaccurate, the consequences can range from costly errors: inefficient shipping routing; damage to offshore equipment—to more profound risks such as underestimating storm intensities, failing to predict hazardous marine conditions, misallocating emergency resources during severe weather events, or even misguiding climate adaptation strategies. These issues directly impact sectors such as emergency management, offshore wind and oil operations, maritime shipping, coastal infrastructure planning, and insurance. Accurate weather and climate models are also critical for agriculture, aviation, and environmental monitoring. In a world increasingly impacted by climate extremes, even small inaccuracies in surface flux representation can cascade into significant errors across these systems, compounding risks and reducing resilience across vital industries.
In this case study we highlight patterns that helped us and our collaborators at NCAR understand when this unexpected behaviour occurs.
One way of visualising the patterns that the Discovery Engine finds is with violin plots, like these. Here, each violin represents the distribution of a single variable, shown on the y axis, under different sets of conditions (or rules) extracted by our system, shown on the x axis. The horizontal dotted lines show the mean of each distribution.
Pattern I
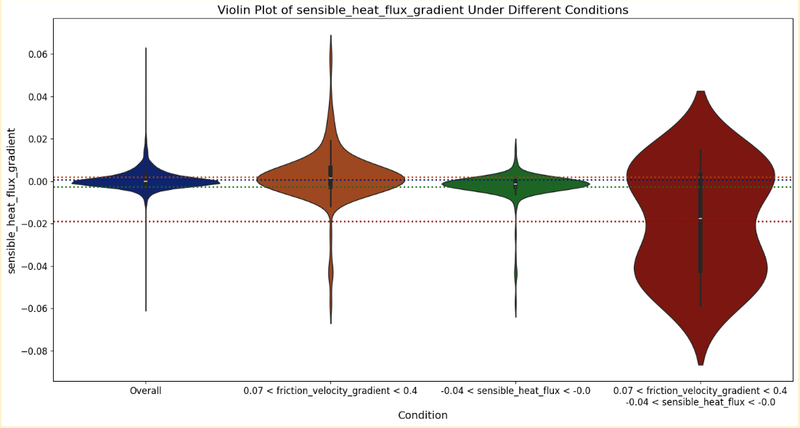
Low heat flux and high friction velocity gradient, signifying stable conditions, are predictive of a negative heat flux gradient. This is not necessarily a violation of the Surface Layer Hypothesis—which permits weak gradients in stable stratification—but it is still surprising. According to foundational theory (Arya, 2001), heat and momentum fluxes are generally expected to be closely aligned in direction and structure due to their shared dependence on turbulent transport processes.
The Discovery Engine showed that these two conditions—when occurring independently—have minimal effect on the mean heat flux gradient. However, when they appear together, the mean is significantly reduced. This kind of interaction is extremely hard to find with manual analysis on large datasets, unless you already know what to look for.
This finding points to an under-appreciated interplay between mechanical and thermal turbulence generation. The implication is that even in conditions where the Surface Layer Hypothesis is technically not violated, its practical assumptions—such as constancy or uniformity in fluxes—may still lead to oversimplifications in models. This reinforces historic calls in the literature (e.g., Beljaars & Holtslag, 1991) to improve turbulence modelling by accounting for coupled behaviours among boundary layer variables.
Pattern II
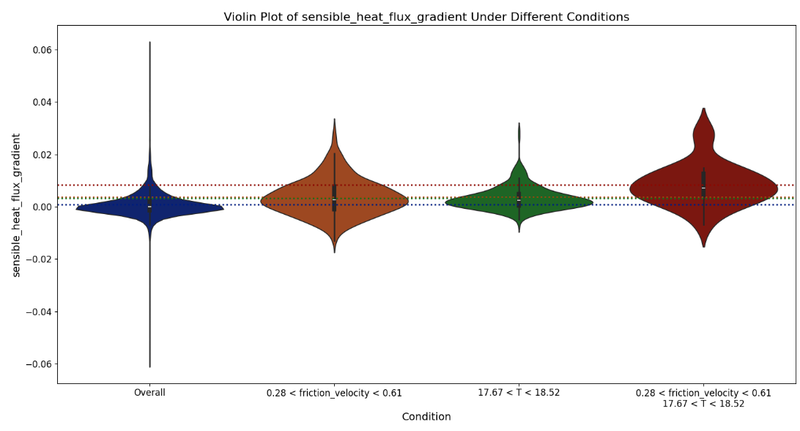
The Discovery Engine found that high friction velocity gradient and low temperature are associated with a high heat flux gradient. This finding is surprising: high friction velocity typically indicates unstable conditions, which are associated with vigorous vertical mixing and constant fluxes through the surface layer. Yet, when paired with low atmospheric temperatures, we observe the opposite—pronounced vertical variability in heat flux.
As Mahrt (1998) has shown, turbulence in cold, shear-dominated flows can be highly intermittent, leading to unexpected behaviour in flux gradients. Our finding suggests that strong mechanical turbulence is not always sufficient to sustain expected levels of heat transport under cold conditions. Instead, cold air may inhibit vertical transfer despite the presence of friction-generated mixing. This challenges assumptions in surface layer theory that presume monotonic improvement in mixing with increased turbulence (Stull, 1988).
Pattern III
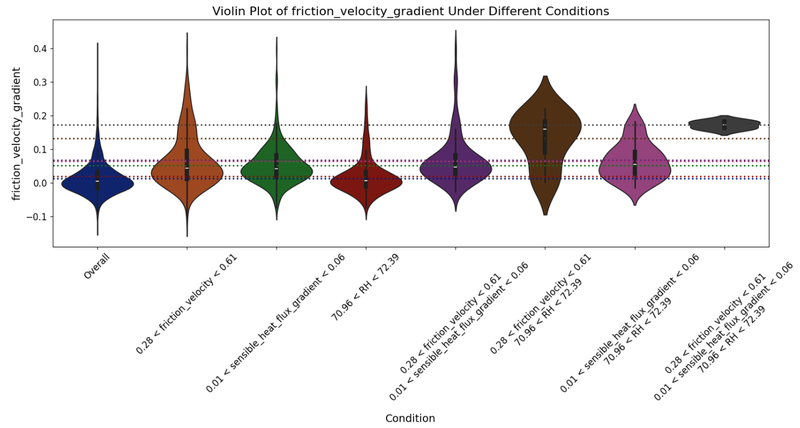
High friction velocity and high relative humidity are associated with extreme friction velocity gradient values, even more pronounced when the heat flux gradient is also high. This contradicts the Surface Layer Hypothesis, since high friction velocity and high relative humidity are characteristic of unstable conditions—under which fluxes are expected to remain constant with height.
When the air is very humid and turbulent, the atmosphere becomes far less predictable. The fact that these conditions lead to more erratic momentum flux means existing models are likely oversimplifying how clouds and storms develop, particularly in coastal and tropical regions. If you're modelling hurricane formation, getting this kind of interaction wrong could lead to substantial errors.
Moisture affects buoyancy generation, especially when coupled with strong winds, yet traditional models often treat its influence as secondary. Work by Kaimal & Finnigan (1994) shows that humidity significantly modulates convective processes and vertical transport. Our findings confirm this, and go further to suggest that moisture has an important relationship with both heat and momentum transfer.
Pattern IV
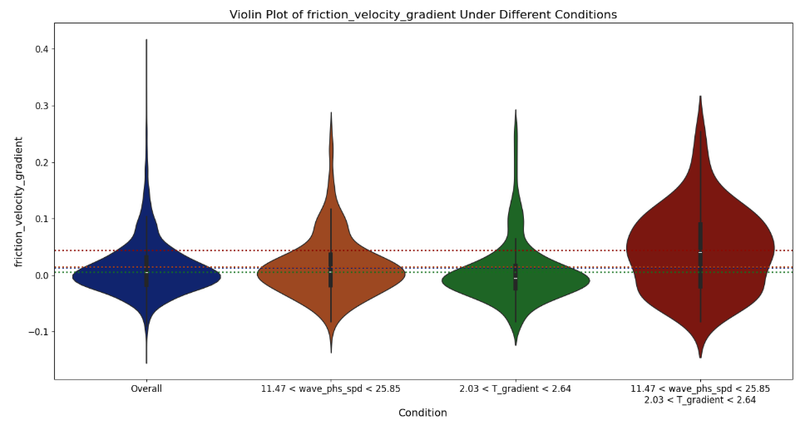
High wave phase speed (the speed at which individual wave crests travel across the ocean surface) and high temperature gradient are associated with more extreme friction velocity gradient values—an outcome that is also unexpected under the Surface Layer Hypothesis – again, because atmospheric instability is assumed to promote vigorous vertical mixing and lead to near-constant fluxes.
This reflects dynamic air-sea coupling, where ocean wave characteristics feed back into atmospheric turbulence structure. Faster-moving waves and sharper temperature transitions appear to amplify turbulent spikes in momentum transport.
Janssen (1989) showed how waves influence surface stress and turbulence – our finding supports this, and also highlights a combinatorial effect with temperature. When wave dynamics are combined with thermal gradients, unanticipated increases in momentum flux appear—even in regimes assumed to be well-mixed. Such overlooked interactions contribute to errors in models used for marine forecasting, offshore operations, and climate simulations that rely on simplistic assumptions about turbulence under unstable conditions.
Bonus!
Aside from these novel patterns, the Discovery Engine also confirmed much existing knowledge – and identified a measurement error in wind speed data collection!
A Scientist's Perspective
The NCAR team expressed both surprise and excitement at the insights surfaced by the Discovery Engine. The patterns identified—particularly deviations from the Surface Layer Hypothesis—offered fresh angles for theory testing and model improvement. One key takeaway was the potential to refine or even reframe the widely accepted definition of the surface layer itself.
The implications go beyond academic insight. As Patrick Hawbecker explained, if Discovery Engine findings continue to hold up, they could unlock major improvements in operational weather forecasting by enabling more accurate representation of surface-atmosphere interactions:
“A lot of the problems in our big global weather models is they're using this assumption and it might be wrong. And if we can improve it...all of these things just improve if you make one improvement in the model.”
What makes the Discovery Engine particularly powerful, according to NCAR researchers, is its ability to identify subtle, multivariate interactions that human analysts might overlook. Rather than testing predefined hypotheses, it explores relationships autonomously, surfacing emergent behaviours that challenge long-held assumptions:
“This has been a theory for 60 to 70 years now that nobody's been able to really modify or improve.”
By rapidly surfacing these novel insights, the Discovery Engine accelerates scientific inquiry—offering a new toolset for researchers navigating complex environmental data. As Sue Haupt noted:
“You’re doing a different type of analysis than we would have. I am finding that a fascinating way to go about scientific discovery.”
NCAR scientists are currently collecting more data to feed the Discovery Engine – both to further validate these disruptive findings, and to see what other patterns might lie hidden.
These results underscore the potential of data-driven discovery to surface meaningful, theory-challenging insights. The Discovery Engine not only reproduces known relationships—because it doesn't bake in any assumptions, it's able to answer questions scientists didn't even think to ask.
By revealing where long-held assumptions might fail, these findings open new paths for scientific inquiry. As climate systems grow more volatile and weather predictions become more critical, this kind of flexible, exploratory analysis can help us improve our models—and better understand the planet we live on.