Discovering Hidden Patterns in Plant Biology
Jessica Rumbelow
Co-founder & CEO
We recently partnered with the Montpellier Institute of Plant Sciences to apply the Discovery Engine to a tough, high-dimensional problem in plant biology: how do genotype and environmental conditions shape early root architecture in Arabidopsis thaliana?
If you’ve ever studied plants, you’ve met Arabidopsis. It’s the go-to model organism in plant biology—fast-growing, genetically tractable, and simple enough to serve as a window into more complex crop species. In this study, we focused on its root system during the first 16 days of growth, a critical period when root architecture begins to define how efficiently the plant will access water and nutrients.
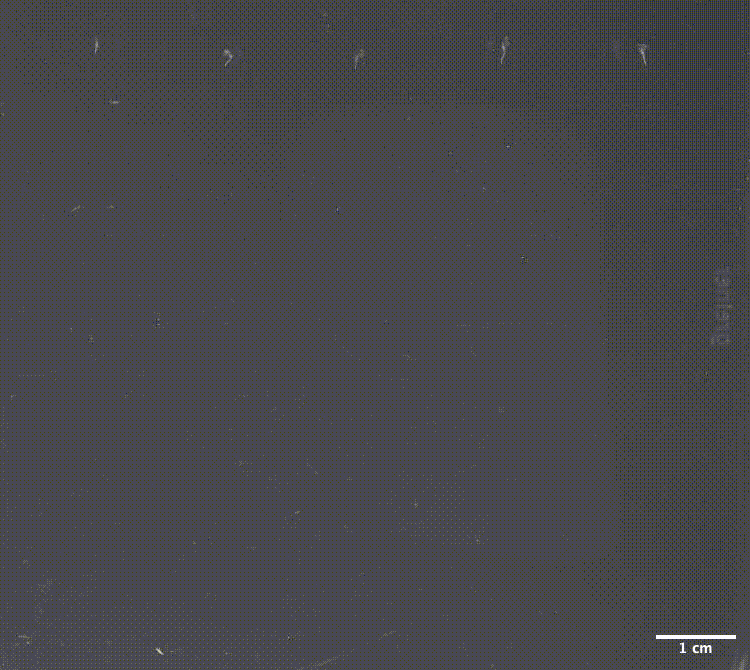
Why this matters
Root architecture isn’t just an academic curiosity. It directly influences drought resistance, nutrient uptake, and ultimately, crop yield. Understanding how roots develop under different genetic and environmental conditions could transform agricultural practices—especially as we face increasingly unstable climates.
The dataset included variables like days since planting, CO₂ levels, room temperature, sorbitol (a proxy for water stress), genotype (20+ variants), and nutrient treatments (50+). The challenge scientists face is finding combinations of these inputs that optimise either total root length or alpha—a metric that reflects how a plant trades off between efficient transport and energy conservation in its root structure. We also looked at scaling distance to the front, a parameter that captures how close a plant’s actual growth is to its theoretical optimum between efficient transport and energy conservation.
We fed this data to the Discovery Engine, which extracted many patterns describing relationships between these variables. Let’s walk through three of the findings that that our collaborator found most interesting—insights that weren’t apparent using conventional methods.
One way of visualising the patterns that the Discovery Engine finds is with histograms, like these below. Here, each colour represents the distribution of a single variable, shown on the x axis, under different sets of conditions (or rules) extracted by our system, shown on the legend. The vertical dashed lines show the mean of each distribution.
Pattern I: Synergistic Effect of Wild Type Genotype and Moderate Nitrogen on Root Length
This is important because total root length determines how far a plant can reach for water and nutrients—critical for growth in dry or nutrient-poor environments. It represents how much material the plant invests in its root system.
The Discovery Engine identified a synergistic interaction between the Wild Type (WT) genotype and a mild-nitrogen nutrient condition (N11400_550, where the plants were grown for 5 days under 11 400µM, then for 12 days under 550µM), leading to a significant increase in total root length. Individually, the WT genotype and the high nitrogen condition increase root length somewhat – but together, the effect is much more pronounced.
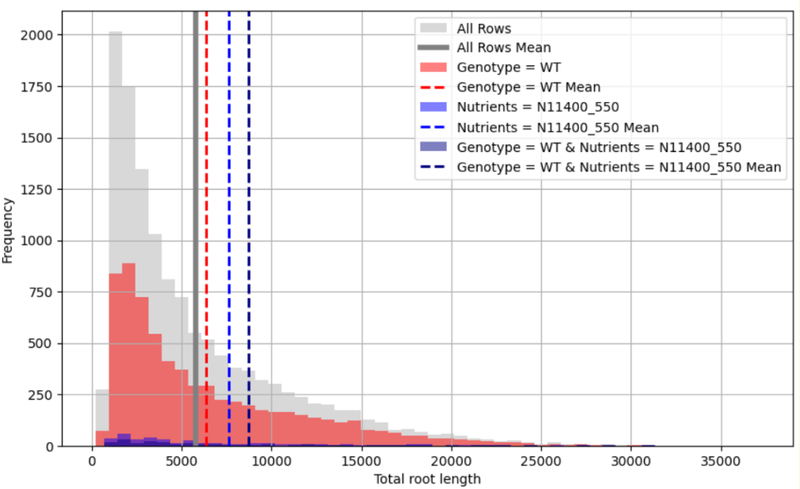
This property was not previously known! But, it makes sense – it's supported by literature indicating that nitrogen availability modulates root system architecture by triggering a root foraging response (Gruber et al., 2013).
This is exactly the type of insight that's hard to find with standard data analysis, unless you look at every possible combination of every variable (often prohibitively computationally expensive), or already know what you're looking for.
Identifying this kind of interaction offers a pathway for improving root system performance in crops by tailoring both genotype and fertiliser inputs. It demonstrates that simply increasing nutrient levels or selecting for a strong genotype in isolation misses more powerful combinations.
Pattern II: Unanticipated Alpha Increase in hy5/nrt1.1 Genotype Under Nutrient Deprivation
Typically, when nutrients are scarce, plants minimise energy expenditure on root growth, which reduces their alpha value—a measure of how much they prioritise energy efficiency over ideal nutrient transport structures.
But here’s the twist: the hy5/nrt1.1 double mutant increased its alpha even under nutrient-free (mock) conditions. That means it maintained a more complex root system—favouring better nutrient transport—despite the lack of resources. This effect was most pronounced at day 7 of growth.
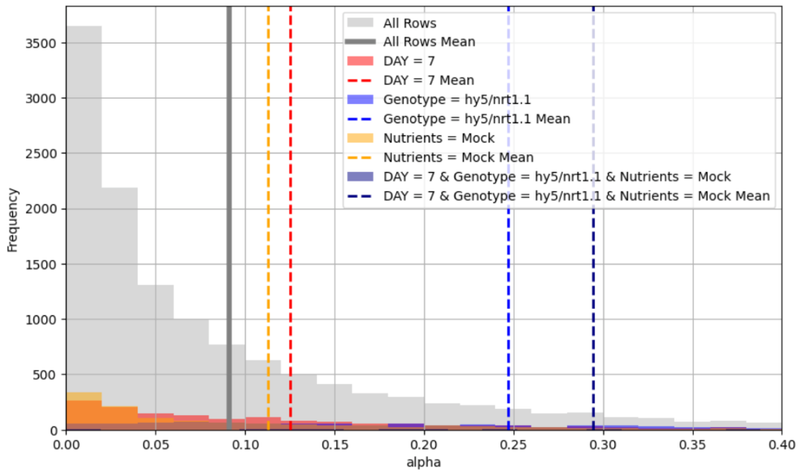
This is exciting because it implies a form of resilience built into this genotype that was not previously understood. From an agricultural perspective, such genotypes might be better suited to poor soils or degraded environments.
What could be behind this behaviour? HY5 is a transcription factor—a protein that binds to DNA and regulates the activity of other genes. In plants, transcription factors like HY5 help orchestrate responses to environmental cues, such as light, temperature, and nutrient levels. When HY5 is disrupted, as in this mutant, the plant's usual regulatory responses to stress are altered.
Paired with the NRT1.1 mutation, which affects nitrate sensing, the result is a plant that ‘decides’ to grow a more complex root system even when nutrients are scarce. This suggests the plant is following a different set of internal rules—potentially sacrificing short-term efficiency in favour of long-term adaptability. Recent research supports this hypothesis, showing that the HY5–NRT1.1 interaction plays a central role in how plants integrate temperature and nutrient cues to direct growth (Lee et al., 2024).
This novel finding points to a valuable class of stress-resilient genotypes that maintain high transport capacity even under nutrient deprivation, providing a target for genetic engineering or breeding programmes aimed at increasing tolerance to poor soil conditions. It also challenges assumptions about energy trade-offs under scarcity, offering a new way to think about “efficiency” in plant systems—especially in the context of climate change and declining soil fertility.
Pattern III: Manganese, Without Phosphorus, Enhances Root Architecture Efficiency
In this pattern, we saw that the absence of phosphorus (P625_0) and moderately high manganese (Mn50_10) helped reduce scaling distance to front. This means the plant’s growth was much closer to what theory predicts as “optimal”—a structure that balances energy cost with efficiency of water and nutrient transport.
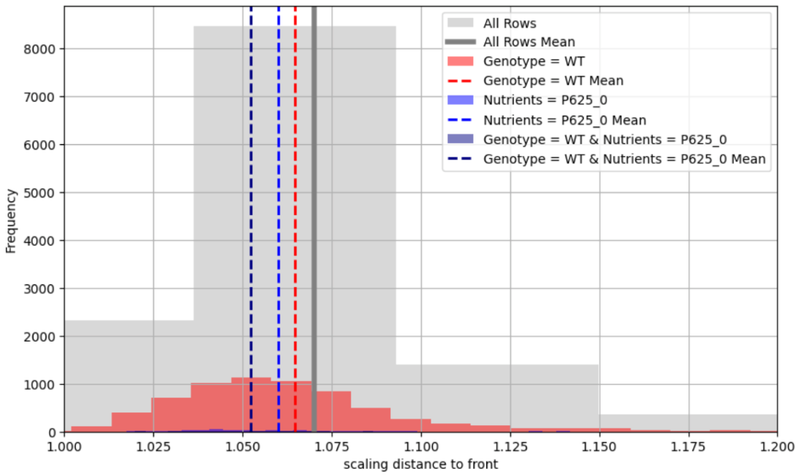
Why does that matter? Because in agriculture, we don’t just want plants that survive—we want plants that use their resources wisely. A plant that builds a root system close to its theoretical optimum can grow faster, withstand stress better, and produce more yield per unit of input.
The effect of this combination was unexpected, but is supported by existing knowledge. Phosphorus is vital to several biological functions—it’s part of ATP (the energy currency of the cell), DNA and membranes. When phosphorus is scarce, plants adapt by developing more extensive root systems to forage for it. This is well-documented in model species like Arabidopsis and crops like maize (Williamson et al., 2001). Manganese, meanwhile, is involved in photosynthesis and enzyme activation. While not typically highlighted in root studies, it plays an indirect role by supporting the overall metabolic processes that enable growth. Moderate enrichment has been shown to improve root strength and stress tolerance in some crops (Zhang et al., 2024).
For plant science, the Discovery Engine enables a more strategic approach to nutrient supplementation: not just applying fertilisers broadly, but understanding how specific nutrient combinations fine-tune architecture, and how they interact with different mutations. Identifying these non-linear effects would previously require months of manual analysis – but now, we can find them automatically in hours.
A Scientist's Perspective
Our collaborator Matthieu Platre described the experience of using the Discovery Engine as both surprising and validating. It returned many patterns that aligned with well-established knowledge, and others, like those above, that point to completely novel findings.
In one instance, the model highlighted a condition he had accidentally excluded from an earlier analysis—one that later proved to be highly significant. That kind of unexpected correction underscored the system's reliability and utility.
He emphasised how the Discovery Engine enabled a more objective approach to hypothesis generation, freeing him from the natural bias that comes with having preconceived expectations. Instead of starting from what he thought might be interesting, he could start from what the data actually suggested.
“I didn't expect that. But I mean this is good, right? That's why you guys are there. To see something that I cannot see.” - Matthieu Platre
He found the automation particularly compelling - we saved him "three months of scrolling in Excel". Beyond just accelerating analysis, the Discovery Engine is actively reshaping how he designs his experiments. Platre has already changed his setup for a major European Research Council (ERC) grant based on our recommendations, using incremental nutrient concentrations to maximise the system’s interpretive power.
Importantly, he sees this not just as a personal productivity tool, but as a capability shift for the field. The volume and variety of traits in root system architecture are overwhelming, and conventional tools can’t keep up. “You are dealing with one root system architecture and a tremendous amount of traits,” he explained. “Plasticity is key for plant adaptation, and AI tools that can assess that could bring something really cool.”
Looking ahead, he’s planning a large-scale study involving nine nutrients at four concentrations each—a complex combinatorial problem. Here, he sees the Discovery Engine as critical for understanding his results.
This case study highlights what makes the Discovery Engine powerful: saving researchers months of manual analysis, and answering questions they didn't think to ask.
If you're working with complex biological data and want to to see what you're missing, let's talk.
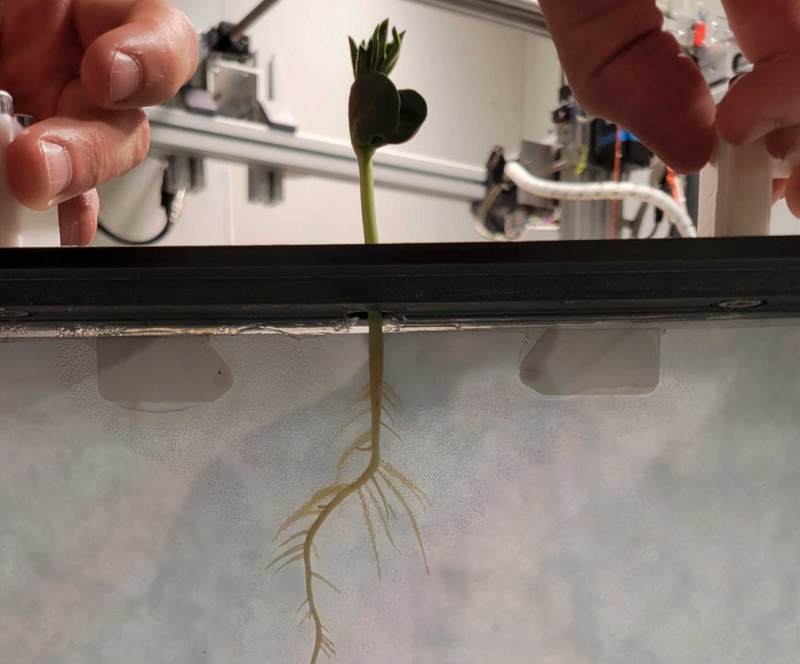